Bottom Line Up Front:
Winning the undersea fight requires robust acoustic surveillance.
Waiting for conflict will be too late. Effective AI-enabled surveillance capabilities require data that must be collected now.
Traditional submarines and small autonomous vehicles will coexist in the future subsurface environment. To train effective AI models, datasets need to include the widest possible variety of targets.
The still scenes of the ocean below the surface seem to be a quiet and peaceful place with soothing white noise. But this soothing quiet is turned into a cacophony by all forms of human maritime activity – and a navy that can effectively detect this activity will have an enormous warfighting advantage. The challenge is making sense of the noise. This problem’s difficulty is dramatically increased by the myriad of small, electric unmanned surface and autonomous underwater vessels that stand ready to prowl contested waters. Fortunately, as the development of seemingly signatureless unmanned watercraft has advanced, so too has the means to detect such threats.
Recently, undersea sensor packages equipped with artificial intelligence (AI) have been developed with the singular purpose of cutting through ambient noise to locate and identify adversary maritime threats. However, AI does not create itself, but rather relies on massive amounts of data to train models. Like a pre-season game hunter setting up trail cameras, an extensive network of acoustic sensors must be proliferated to establish a baseline pattern of activity and data library for AI model building. In this article, acoustic sensor employment considerations and the technical constraints of AI models are presented as key motivators to establishing sonar networks as soon as possible.
SOSUS Legacy Echoes through Time
The US Navy has been capable of listening to adversary naval contacts for decades. During the Cold War, the Sound Surveillance System (SOSUS) could detect and locate numerous undersea targets and anomalies. For example, in the 1960s, the SOSUS community detected the sinking incidents of the USS Thresher, USS Scorpion, and the Soviet K-129. The SOSUS originally focused on detecting Russian subsurface threats transiting the Greenland-Iceland-UK gap, but proved valuable in other areas of operations. While the methods were classified at the time, we now know the operators and analysts in the SOSUS network relied on “LOFARgrams” for crucial acoustic information. Low-Frequency Analysis and Ranging (LOFAR) grams, like the one shown below, used a combination of black bars and spaces to represent acoustic sounds.
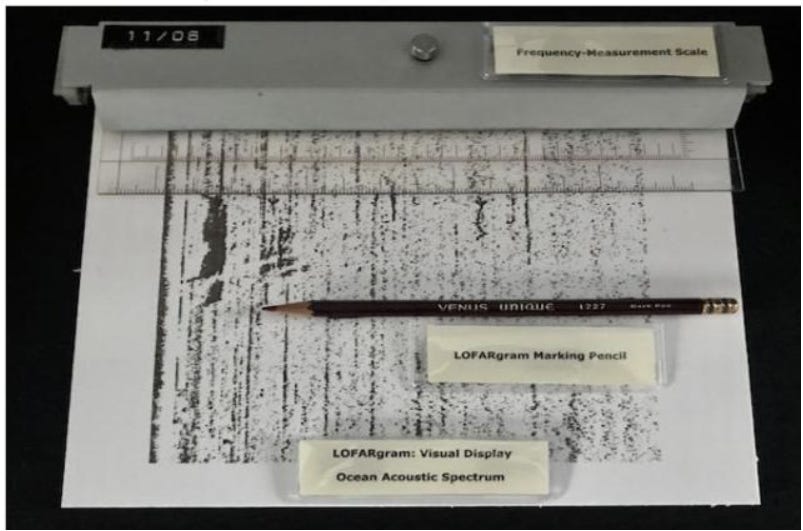
Fixed sensors in the SOSUS network transmitted acoustic collections to listening stations where operators printed the LOFARgrams on large spools of paper. Exploiting LOFARgrams for intelligence purposes was an arduous human task requiring analysts to carefully interpret the abstract markings on these spools of paper and apply subject matter expertise to identify anomalies. In addition to these time and labor intensive exploitation requirements, the SOSUS network had widely known fixed locations, making it unsuitable for future conflict.
Today’s AI models1 such as neural networks also render such manual interpretation of acoustic detection data obsolete. When properly trained, AI acoustic detection models outperform humans at an astonishing rate. Advanced AI-enabled software packaged in a hardened, versatile, and mobile form factor is table stakes for the next generation of acoustic sensor networks, whose chief advantages include global deployability, wireless communications, and foundational AI algorithms that will be responsible for identifying acoustic anomalies. Similar AI models developed for hobby applications like the Merlin Bird Identification phone app demonstrate the type of inputs required to build such models, and how these models can be operationalized.
What We Can Learn from Bird Watchers
Merlin Bird ID, the popular bird watching phone app from Cornell Lab, gives us a glimpse of the functionality, data requirements, and software that are foundational to acoustic collection networks. The application helps users identify birds by their calls using a specially trained model that predicts a bird’s species from user-submitted sound recordings. While the underlying model is invisible to the user, the application processes and interprets the recording input like an AI version of a SOSUS analyst. When a user uploads a sound recording, the recording is converted into a visual representation of sound waves over time called a spectrogram, a closely related cousin of the LOFARgrams created by SOSUS. The spectrogram serves as the primary input to the application’s AI model, which analyzes the spectrogram for anomalies and outputs the probability that the presented sound originates from a specific bird species.
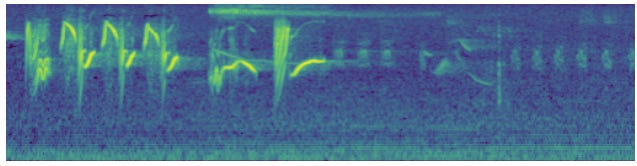
The Merlin Bird ID is powered by a deep convolutional neural network model called Sound ID. As asserted before, high quality data for AI model training is foundational to performant neural networks. Without good data, these models are subject to the “garbage in, garbage out” principle. By extension, without quality undersea data, AI-enabled undersea acoustic sensors will miss threats and create exploitable situational awareness gaps. According to the Sound ID website, Cornell Lab claims that Merlin Bird ID can correctly identify 458 different birds, but only after training the underlying Sound ID model on 266 hours of unique bird calls and environmental audio data. In an ideal world, sensors like Anduril’s Seabed Sentry or Optics11’s OptiBarrier would boast similar identification capabilities, correctly identifying every surface and subsurface threat that an adversary operates. However, the time, labor, and resources required to collect, tag, and aggregate 266 hours of threat acoustic signatures will pose an extreme challenge. The next generation of stealthy autonomous unmanned vessels like the Chinese HBU-001 and Anduril’s Copperhead AUVs will project less noise into the water column, resulting in smaller acoustic signatures. Furthermore, the challenge of detecting quiet undersea threats becomes impossible if acoustic sensors are not emplaced and exploited before competition turns into conflict.
Layered, Deployable, and Connected Networks
Undersea surveillance networks must be layered, deployable, and connected to ensure actionable information supports timely defense operations, and several recent industry innovations indicate an acknowledgement of this reality. For example, European university researchers have recently tested a new sensing technique called Distributed Acoustic Sensing (DAS) that utilizes existing fiber optic sea cables to detect seismic and acoustic signatures. The DAS technique also benefits from near real-time reporting, since the sensors are the cables themselves and are therefore already low-latency and hard-wired to a shore station.
Some fiber optic sensing companies like Optics11 have advocated that new defense-focused fiber optic cables should be emplaced around routinely targeted undersea infrastructure like the Baltic Sea data communication cables. Optics11’s fixed seabed monitoring system, OptiBarrier, advertises a hydrophone-based system that can detect the complete array of undersea threats from divers to submarines. OptiBarrier breaks effective system ranges into three envelopes with associated threat detection objectives from <5km, <30km, and >100km.
Lastly, the aforementioned Seabed Sentry is an AUV-delivered sensing option that advertises active and passive sonar, months-to-year persistence, and an acoustic communications relay capability. None of these systems adequately address the undersea sensing gap on their own, but layered together, both fixed and mobile sensing options start to address the challenge.
For example, layering fixed and mobile undersea sensing assets in a Baltic undersea infrastructure defense scenario could achieve redundancy, deployability, and persistence. To illustrate this concept, the minefield planning simulation from the Damn the Torpedoes: Series on Naval Mining Operations is repurposed to model an acoustic sensor field that collects in the vicininty of the undersea cables connecting Helsinki and Tallinn. In the figure below, fixed systems are depicted as the primary protective assets while mobile systems like the Seabed Sentry are conceptualized as flexibly deployed systems.
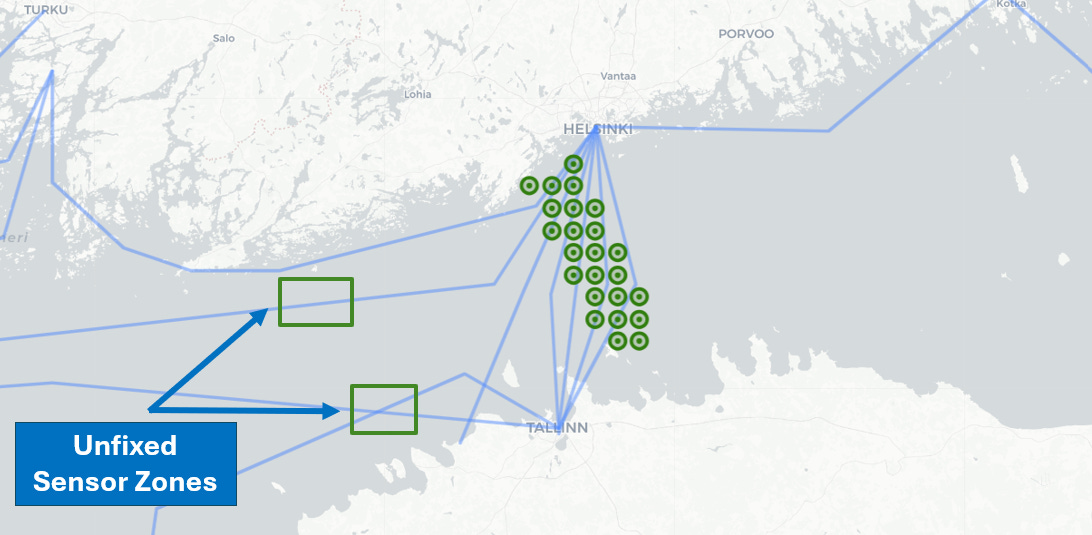
This scenario anticipates integrating systems similar to the Optics11 OptiBarrier with a cable-based DAS capability to establish a persistent and fixed sensing capability. Fixed systems will also provide the foundational AI model training data that will ultimately enable more complex threat detection models. Meanwhile, mobile systems should provide flexible surveillance options and be leveraged to enlarge or target specific collection areas and events. Much like the SOSUS, the locations of fixed assets established near critical infrastructure will be known and avoided by adversarial actors in the region. Therefore, unfixed assets must be creatively employed to collect and survey the other areas of interest.
Sense and Make Sense First
Undersea acoustic surveillance is not a luxury or grand advantage in today’s competition or tomorrow’s conflict. The next conflict will integrate exquisite autonomous platforms that evade traditional detection. Only by deploying and training layered, AI-enabled acoustic sensor networks now can we ensure our forces are prepared. The SOSUS proved the value of undersea surveillance and continues to provide foundational lessons to the community. The lesson today is clearer still: deploy late and your network is worthless.
Victory will belong to those who sense and make sense first.
Read More of Our Work Here:
Rearmament Along the Rhine: Part 1 of a Series on Rebuilding the German Military
Precision Mass at Sea: A Preliminary Analysis
Gone MAD: How the Golden Dome Threatens the Logic of Nuclear Deterrence
One Million Suicide Drones with Chinese Characteristics
The views and opinions expressed on War Quants are those of the authors and do not necessarily reflect the official policy or position of the United States Government, the Department of Defense, or any other agency or organization.
For the purpose of this article, AI, AI models, and neural networks are used interchangeably.
Integrated AI-enabled undersea sensing network in the first island chain when?